Thinking at a Landscape Scale: Part 2
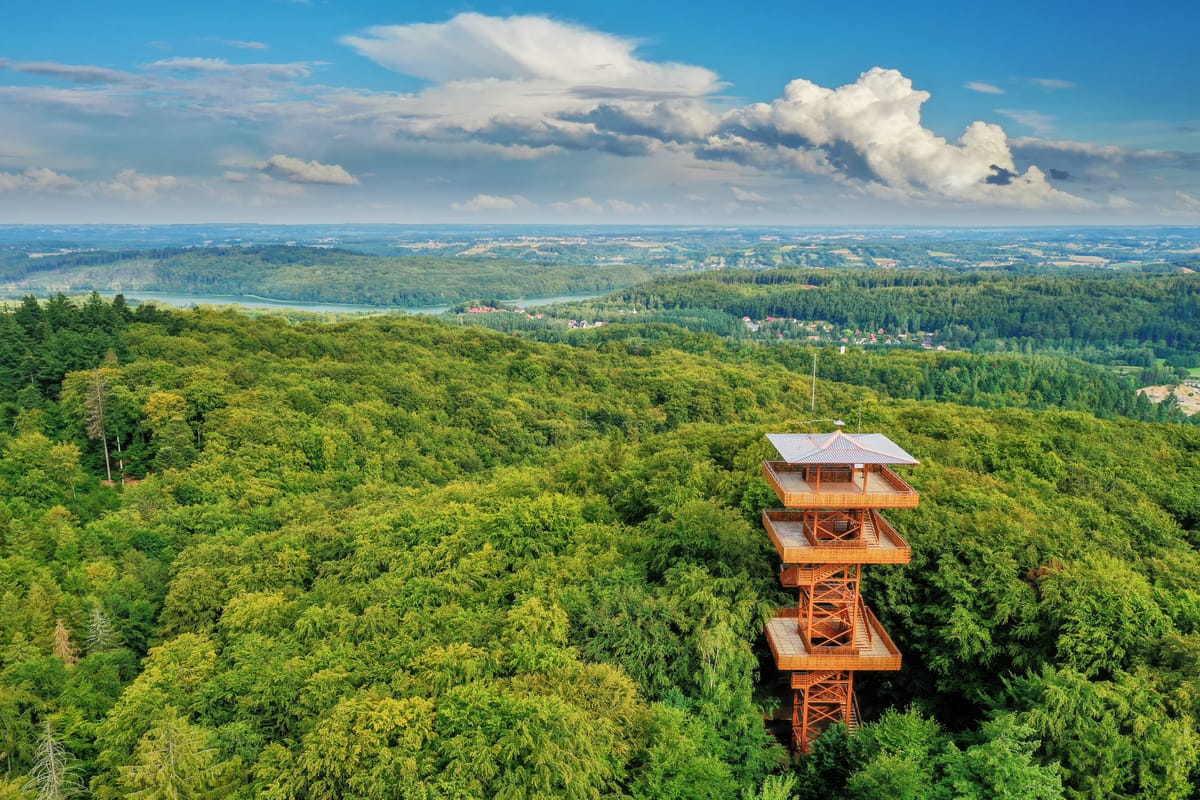
Autonomous eco-labs
Following the intro in Part 1, I want to state more directly the progression I am trying to describe:
- The capacity for cheap hardware and distributed computing will enable continuous, inexpensive, global ecosystem monitoring.
- We need to work through ways to make it ethical, accessible, understandable, and useful (however we want to define that last point).
- One of the most important implications of accessibility is that it has the potential to enable greater numbers of more diverse, underrepresented thinkers and makers to solve problems that matter to them, not necessarily some individual institution.
Beyond the technological building blocks mentioned in the previous post, we already have structures in place to deploy that tech - let's start with institutions. The following list of observatory networks is most certainly only a fraction of the total:
- The U.S. National Weather Service and local, regional, and international analogs
- The Integrated Ocean Observing System (IOOS) and its international counterparts
- The U.S. Department of Energy's AmeriFlux and Atmospheric Radiation Measurement (ARM) facilities
- The California Irrigation Management Information System (CIMIS) and OpenET networks
- The USGS Streamgaging Network
- The Californa Department of Water Resources system data
- Innumerable private weather data, telemetry, and RTK networks
Now try using them all together, or mix and match a few, and you'll know that the availability of building blocks and the organizations to house them is not enough. Dumping petabytes of data on a website is not enough. We still have a long way to get to true sensemaking.
But now contrast this abundance of unruly earth system data with what we have for living systems. The potential of automated biodiversity surveys was the focus of a 2019 paper by Justin Kitzes and Lauren Schricker (hereafter K&S). Early on, the authors issue a stark statement: "We have fewer data than we think." This is illustrated by a fantastic graphic:
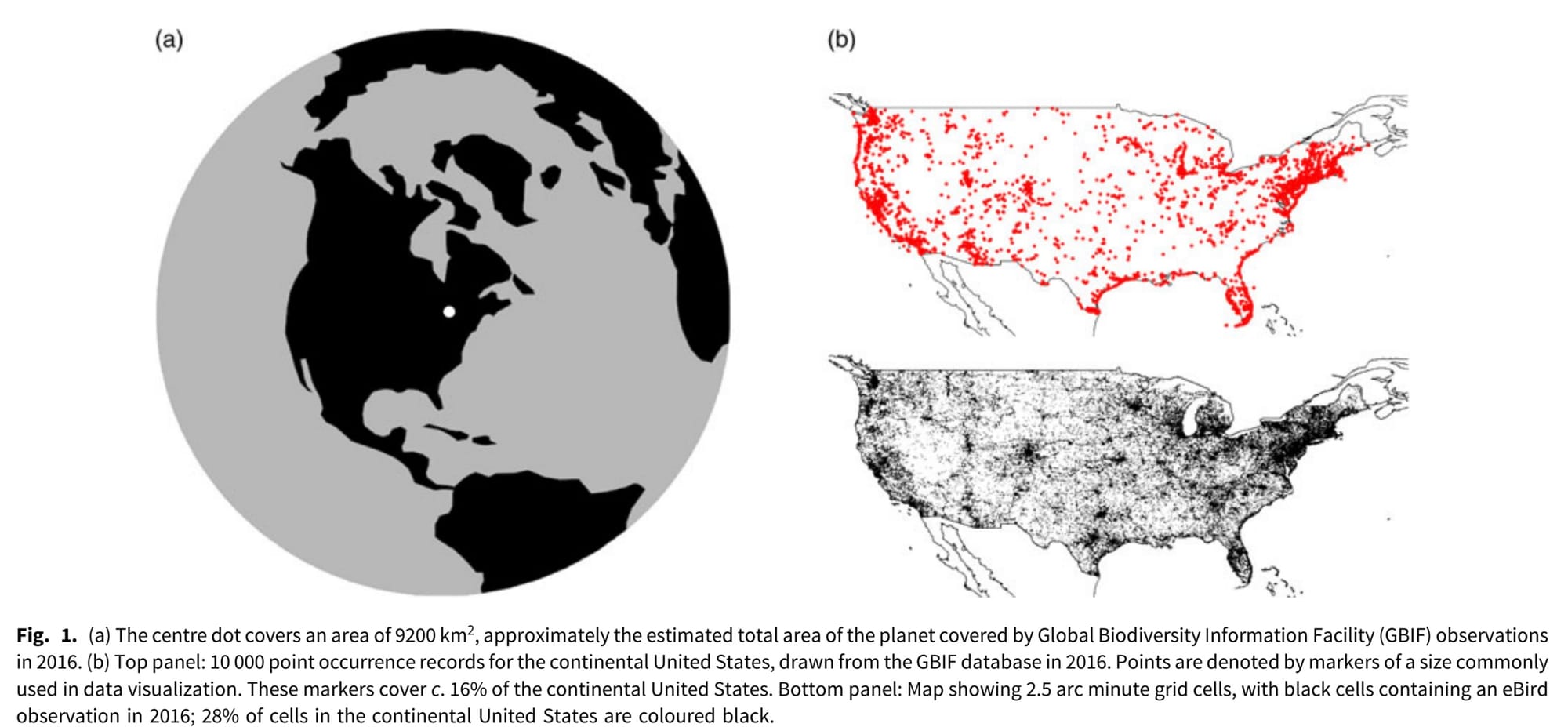
It reminds me of this classic Google map showing the locations of pubs in the UK:
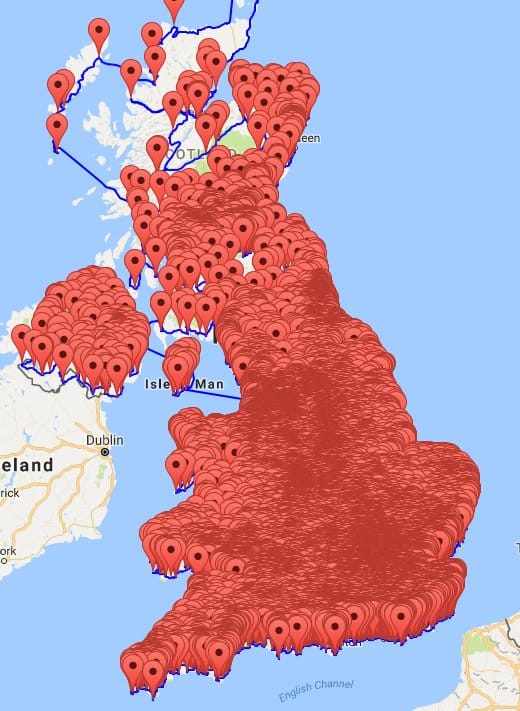
These figures show how misleading "pins on a map" can be. The fact that we can make useful inferences from data that cover only 0.002% of the earth's surface is a tribute to human ingenuity. The point of this essay is not to minimize the insights that have gotten us this far, rather it's a rallying cry for massive new investment in not just more sensors, researchers, etc., but also in making ecosystems more understandable to more people.
On the "sensor" side, the implications of automating biodiversity (more broadly, ecological) monitoring are compelling. From the same K&S paper:
For example, in 2017, the North American Breeding Bird Survey (BBS) (USGS 2017), one of the largest systematic avian biodiversity surveys in the world, surveyed 2646 road transects in the USA, each with 50 stops and a 3-minute point count at each stop. This represented a total of c. 6600 hours of sampling effort. A set of 50 AudioMoth field recorders, purchased for less than US$2500, can equal this sampling effort with a single field deployment [Emphasis added].
And they can stay deployed for months at a time before the batteries need to be changed.
Another way of looking at it is the cost per data point. For perspective, the minimum wage in Oregon is currently $14.70, so if that 2017 survey had taken place here, the data collection alone would have cost at least $100,000. Simple arithmetic suggests that automation in this case could have borne a ~40X improvement (I have done similar hypothetical comparisons using insect studies in tree fruit and estimated the improvement from using electronic monitoring traps to be 53X, so we're on a similar magnitude). If cheaper monitoring yields a 40X increase in the size of that dot from the K&S paper, you have an area the size of Montana, which I would consider a dramatic improvement.
From LOCs to SSOCs
It's important to note that we do have ecological observatory networks, such as the National Ecological Observatory Network (NEON) and the Long Term Ecological Research Network (LTER), both funded by the National Science Foundation. More on those later.
In my previous post I linked to Compound's Shelby Newsad, who raises intriguing ideas about the possibilities arising from biology lab automation. Might lessons learned from fab labs, the maker ethic, and CubeSats show us how to proliferate landscape-scale ecological sensing systems in a similar way?
Building on the well-known concept of a laboratory-on-a-chip (LOC)*, what if we had SSOCs: sensory-systems-on-a-chip? These would support networks comprised of researchers, technologists, citizen scientists, businesses, and activists cultivating observatories and developing sensemaking methods. Massively deployed olfactory sensors replicating canine noses or moth antennae, ears to hear sounds from the subaudio range to hundreds of kilohertz, eyes that can perceive the world as bees and birds see it. Tools to tie into these networks. And what if we do it with the intention of reaping the benefits that come from scale, bringing down the cost to a level where these ecological sensors could be deployed anywhere they were needed? With some of the current generation of sensor packages running in the low four-figure range, that's not going to happen. Even at a few hundred dollars, it won't suffice. I know a lot of people have put in a lot of work and applied for a lot of patents on these technologies. Someone - whether a university tech transfer office or a VC - wants to see a big price tag on these things, but we will never see ubiquity that way.
If we want ecological sensory systems to work, I think we're going to want to do something different than what we've seen with the earth observation-industrial complex I noted at the beginning of this piece. Thinking at a landscape scale is about more than just the physical aspect - governance matters too.
Challenges we want to solve at Resight Labs
I hope I've started to make the case to deploy large numbers of sensors, but there are other opportunities to stretch our abilities, as well as consequences to think through (e.g., e-waste, bad actors). Researchers will need to reevaluate experimental design and survey methods. This raises the possibility of something I've been thinking about for years: what if the data from this new family of instrumentation were research-grade out of the box? Bringing SSOC networks into being will also introduce new computational challenges: we know how to do sensor fusion for, say, navigation technologies, but what about for living systems? How will they support the basics of classifying, informing, predicting? Do we submit to black box methods or is classical modeling still relevant? There are a lot of ecological modeling packages for one problem or another, but as with the earth system data Tower of Babel, integrating them is a completely different matter. I'll share more about what we're working on in this area soon.
[Part 1] Part 2 [Part 3 - coming soon]
* Note: I am neither the only, nor the first, person to write about "x-on-a-chip" ideas. My particular conception came in response to a program called the Market Shaping Accelerator, which launched an effort in 2023 soliciting ideas related to advance market commitments (AMCs) and other so-called pull mechanisms to spur innovation in climate and public health.